Flow Engineering & Designing Efficient Workflows for Competitor Analysis in Real Estate
When competition is fierce, as it is in real estate, strategic insight into the leading competitors and full data can make the greatest difference. Any real estate developer, investor, or consultant depends upon efficient workflows that ease the process of competitor analysis and project evaluation. Therefore, this article breaks down the flow of the AI-powered workflow designed to analyze leading real estate developers and their projects, focusing on the steps and agents involved, and the value that such a course brings with it.
Overview of Competitor Analysis Workflow Process
This involves a multi-step AI workflow in capturing, validating, and structuring information on the best-performing real estate companies and their flagship projects from a given country. Each step in the process is designed to gather specific data points, analyze them, and present relevant insights suitable for decision-making in the realm of real estate. Here are the key stages of the workflow created in FlowHunt.
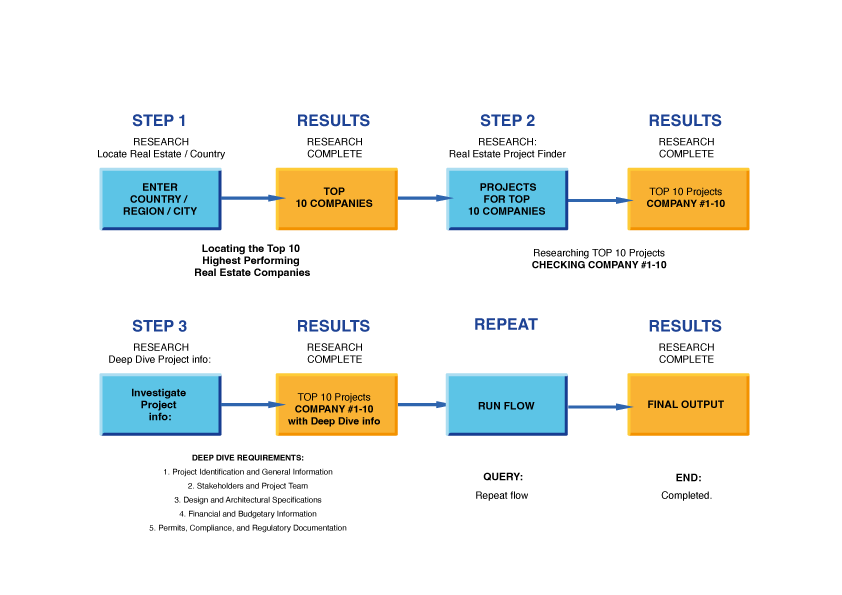
Stage 1: Identifying the Top 10 Real Estate Companies
First of all, an investigation to determine the top 10 performing real estate companies in a selected country is put into operation. Here is how it works:
Enter Country Name – This first input narrows down the entire analysis. For example, if one were to enter “United Kingdom” as the target country, then the AI system would narrow down its research to target companies actively developing large commercial projects in that country.
Top 10 Real Estate Companies:
AI draws from experience in leading real estate companies currently engaged in large commercial projects such as retail parks, shopping centers, office buildings, and mixed-use developments. This process filters out purely residential or small developers and narrows the selection down to only major market players.
Key Data Points:
Real Estate Developer Name – Identifies the name of the company.
Website and Address – Website and location.
Company Size: Gave an idea of the extent of their operations.
This stage identifies verified information about each of the listed developers by utilizing a URL retriever and some of the functionality of Google’s search functions.
Stage 2: Top Projects for Each Developer
When the list of the top 10 real estate companies has been compiled, the next step takes one to delve deeper and find out the key projects of those companies, giving a detailed overview of each company’s key projects.
Project Finder Tool: This AI feature retrieves information about the top 10 projects for each real estate company. For example, if “Company A” is one of the major developers in the country that is being focused on, the AI will run deep analysis to provide information on 10 of its best projects.
Key Data Collected:
Identification and General Information about Projects: This will include project names, type of project-retail, office, mixed use-location, and general objectives of the projects.
Stakeholders and Project Team: Information on primary developers, architects, and contractors.
Design and Architectural Specifications: Layout, zoning, and structural details.
Financial and Budgetary Information: Budget estimates, funding sources, revenue projections.
Permits, Compliance, and Regulatory Documentation: Legal documents, safety certifications, environmental clearance.
Each one of these pieces of information would typically be subject to some sort of validation process for accuracy and applicability, especially compliance-related information and regulatory data.
AI Agents Involved In The Competitor Analysis Workflow
This working process applies the utilization of special AI agents to lighten the work at each step. Thus, here is a breakdown into their respective functions and objectives:
Real Estate Researcher: This agent finds in-depth information on real estate developers, mainly those with large commercial and/or mixed-use projects.
Validator: Checks whether the data being given are of correct criteria, eliminating the irrelevant ones, such as homebuilders; source verification to ensure reliability.
Research Writer: Puts together the research findings into clear, cohesive reports. Then presenting information in a manner that is easy to understand and organize.
Project Researcher: Conduct deeper research on specified projects in order to compile data regarding successful projects and their attributes.
Project Report Writers: Data reporting experts who compile the findings into concise summaries of specific projects.
These agents cooperate through AI-powered tools like Sequential Crew, Self-Managed Crew, and utilities of Google search with the ambition of a well-oiled system working independently.
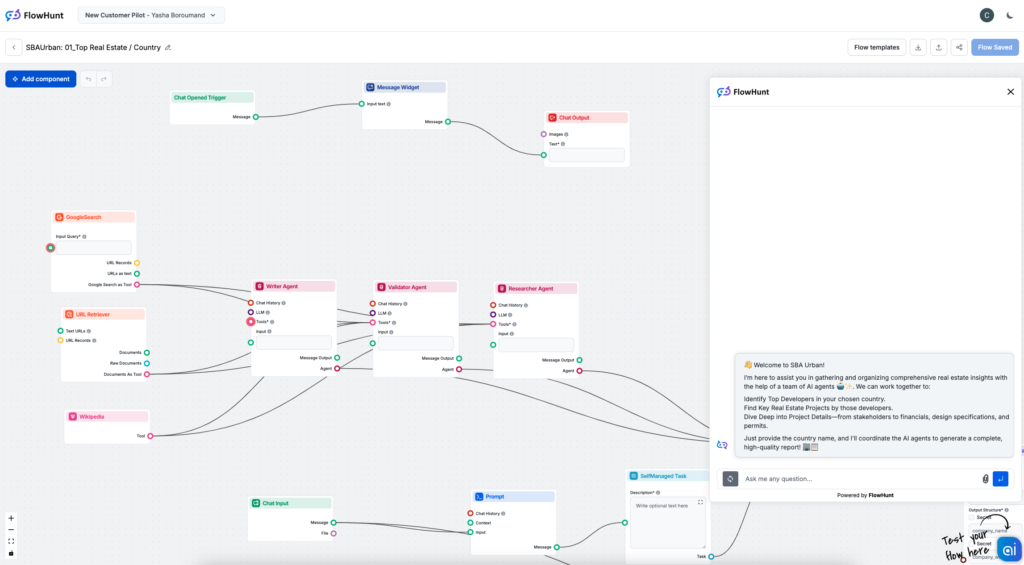
Detailed Project Analysis: In-depth View of Components
For every detected project, the workflow does a piece-by-piece analysis concerning various aspects, including:
Project Identification: official name of the project, the type of project, location, timeline, and general goals of the project.
Stakeholders and Project Team: The collection of information is done regarding key stakeholders involved with the project, including developers, investors, architects, and contractors.
Design and Architectural Specifications: Architectural data is recorded in as much detail as possible, from the lay-out through to structural details and finishes.
Financial and Budgetary Information: The budgets estimated are put in tracking costs, funding sources, and revenue projections that indicate the viability of the project.
Permits, Compliance, and Regulatory Documentation – Building permits, safety certificates, or environmental impact studies are verified for their legality and compliance.
All of these sections are populated by domain-specific AI agents that know source materials relevant to this information and present this knowledge. They use hyperlinks to trustworthy sources so as to ensure verifiability and traceability.
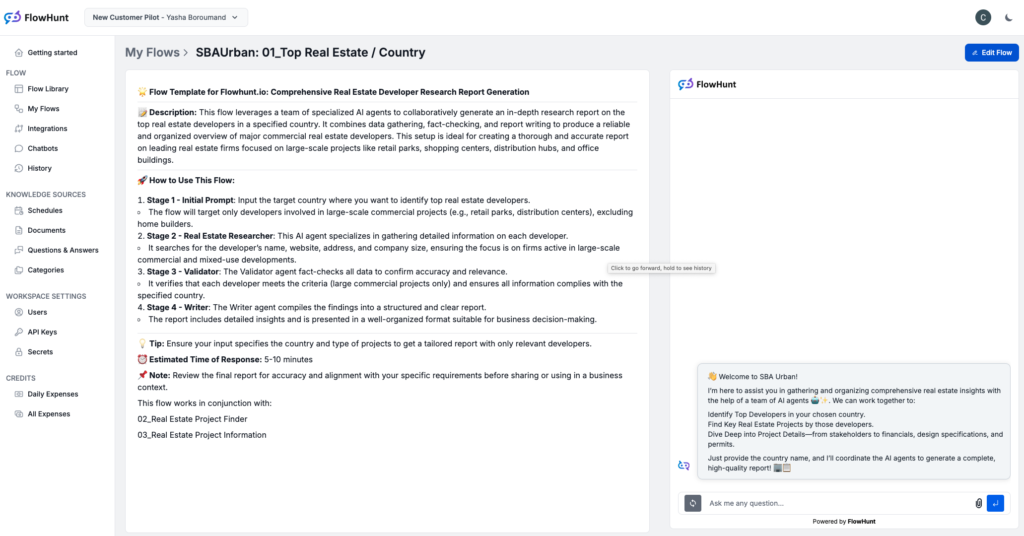
Scaling for Maximum Efficiency
This AI-driven workflow could be extended at various levels of geography and would, therefore, be ideal for those organizations operating at an international scale. The scaling structure could comprise the country level with about 200 countries worldwide, the regional levels comprising an estimated average of 20 regions per country, the city level with about 20 cities per region, and local areas within the cities, in case the city is one of the major urban centers-averaging 10 sub-areas per city.
This exponential structure makes it possible for hundreds of thousands, if not millions, of data points to be embraced in the workflow for a comprehensive competitive analysis of companies with global interests in real estate.
Final Thoughts:
Why This Workflow Offers Value for Money
The proposed AI-driven competitor analysis promises value for money regarding its precision, scalability, and automation. Targeting large-scale, commercially impactful projects and leading developers of real estate, this workflow provides:
1. Employee Savings:
Automates repetitive tasks, freeing employees to focus on higher-value work and reducing labour costs.
2. Enhanced Productivity:
Streamlines operations and integrates with existing tools, boosting efficiency and reducing time spent on projects.
3. Error Reduction:
Minimizes human errors, saving on potential rework and compliance costs.
4. IT and Development Savings:
No-code setup avoids costly custom development, making implementation quick and affordable.
5. Resource Optimization: Ensures teams operate at peak efficiency, reducing operational costs by up to 30%.
In other words, this structured AI workflow saves the much-needed hours and resources. They also equip real estate professionals with the profound insights to make informed, competitive decisions within an evolving market.
Find the Perfect Business Name with AI: A Step-by-Step Guide
Please provide the content of the webpage you would like summarized into a meta description.
Scripted Chatbots vs AI Chatbots
Explore the difference between scripted and AI chatbots to choose the best fit for your needs. Discover their unique advantages today!