Understanding how different models perform on real-world tasks can provide valuable insights for researchers, developers, and users alike. Google’s Gemini 2.0 Flash-Lite represents an interesting development in the push for more efficient AI systems. Let’s explore how this model performs across various tasks based on detailed performance analysis.
Task Performance Overview
The analysis examined Gemini 2.0 Flash-Lite across five key areas:
- Content Generation
- Calculation
- Summarization
- Comparison Analysis
- Creative Writing
Each test reveals different aspects of the model’s capabilities, from logical reasoning to creative synthesis.
Content Generation: Project Management Fundamentals
When tasked with creating comprehensive content about project management, the model demonstrated a methodical approach to research and content creation.
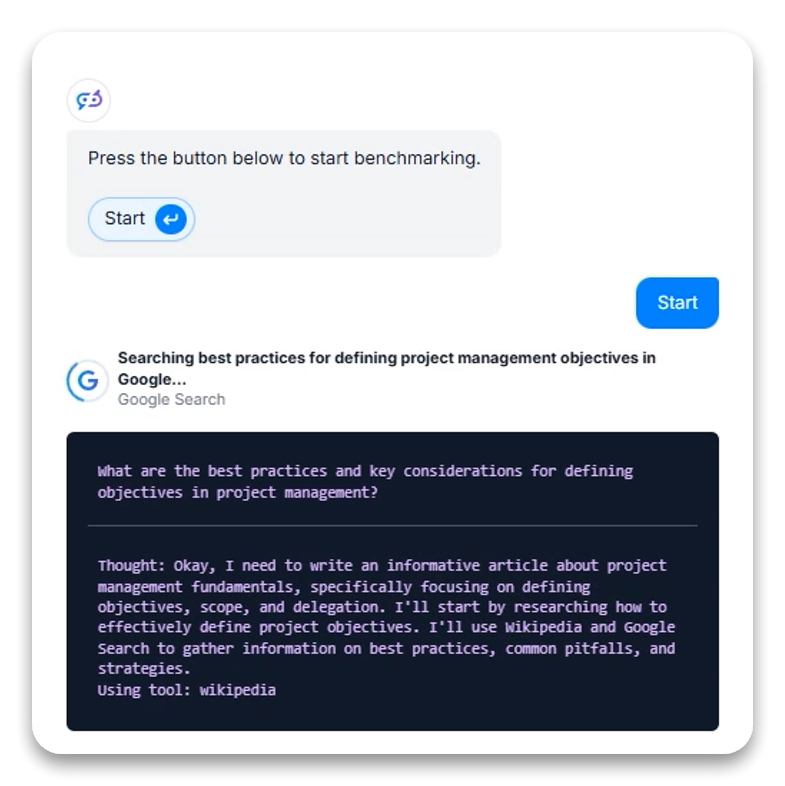
The Process:
- Research Strategy: The model began by using Wikipedia for general context, then refined its search using Google to gather specific information on best practices and pitfalls.
- Information Synthesis: Rather than just compiling information, the model organized findings into a well-structured article with clear sections.
- Comprehensive Coverage: The final output thoroughly addressed defining objectives, explaining the SMART framework, and highlighting common mistakes in project management.
The model completed this task in just 30 seconds, producing content at approximately a 11th-grade reading level – appropriate for professional contexts without being unnecessarily complex.
Calculation: Financial Analysis
Financial calculations offer a clear test of logical reasoning abilities, and here the model displayed strong analytical skills.
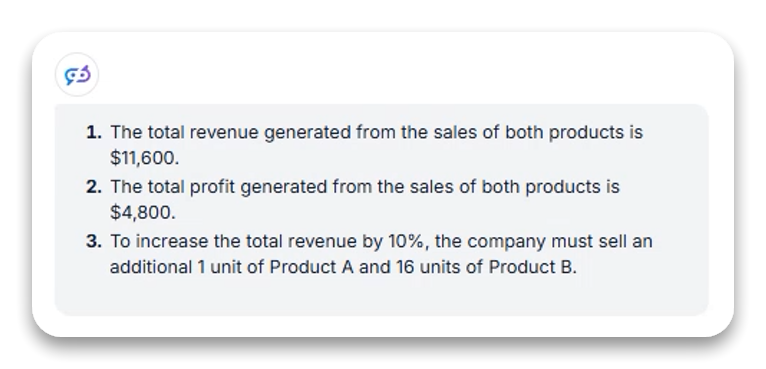
The Challenge:
The model needed to calculate revenue and profit for products with different costs and selling prices, then determine how many additional units would be needed to increase revenue by 10%.
The Approach:
- Logical Decomposition: The model broke down the problem into manageable steps, calculating revenue and costs separately before determining profit.
- Strategic Thinking: When faced with an optimization problem (minimizing additional units while meeting revenue targets), the model explicitly stated its assumptions and prioritized higher-priced products to minimize total units needed.
The calculations were completed in 19 seconds with precise results: $11,600 in total revenue, $4,800 in profit, and a recommendation to sell 1 additional unit of Product A and 16 units of Product B to achieve the 10% revenue increase.
Summarization: Concise Information Extraction
The ability to condense complex information into digestible formats is increasingly valuable in our information-rich world.
Performance Highlights:
- Efficiency: The model summarized a lengthy article about AI reasoning in just 3 seconds.
- Focus and Brevity: While given a 100-word limit, the model delivered a 70-word summary that still captured all key points.
- Content Selection: The summary successfully highlighted the most significant aspects of the source material, including historical context, applications, and recent advancements.
This demonstration of efficient information processing shows the model’s potential utility for research assistance and content curation.
Comparison Analysis: EVs vs. Hydrogen Vehicles
For this task, the model needed to compare two complex technologies while considering multiple environmental factors.
Strengths Displayed:
- Structured Organization: The output was clearly organized under distinct headings addressing energy production, vehicle lifecycle, emissions, and overall comparison.
- Balanced Perspective: The analysis acknowledged the dependencies and tradeoffs of both technologies, such as the importance of electricity sources for EVs and hydrogen production methods for FCEVs.
- Technical Accuracy: The content demonstrated an understanding of the technical aspects of both vehicle types, presented at approximately a 15th-grade reading level (appropriate for the technical subject matter).
The model completed this detailed comparison in 20 seconds, producing 461 words across 30 sentences that effectively addressed all requested factors.
Creative Writing: Future-Focused Narrative
The final task tested the model’s ability to blend analytical thinking with creative expression.
The Process:
- Initial Analysis: The model first created a structured analysis of environmental changes and societal impacts of an all-electric transportation system.
- Creative Synthesis: It then transformed this analytical framework into an engaging narrative set in the year 2147.
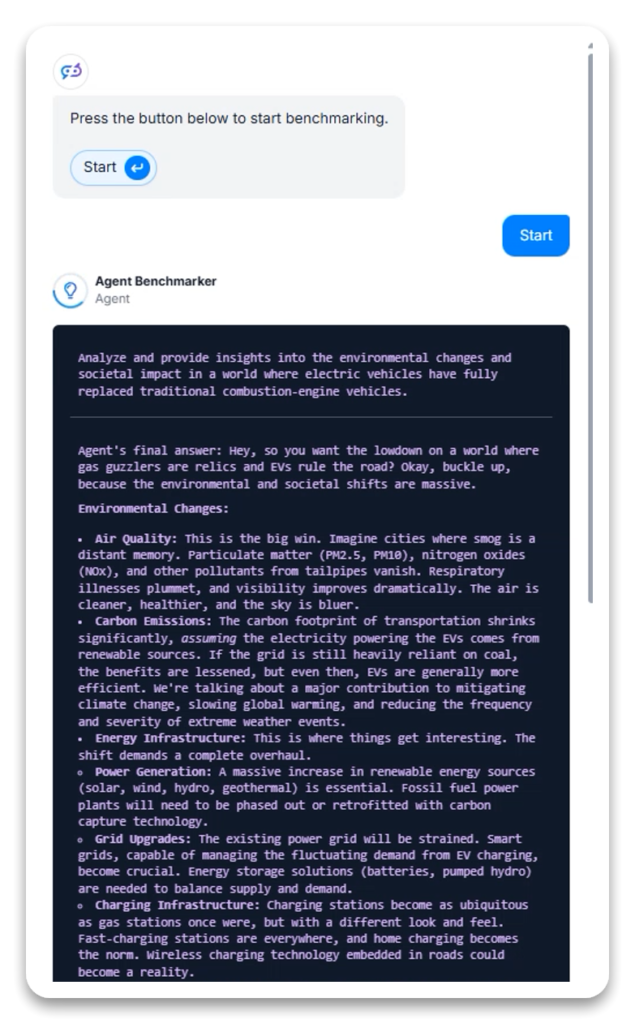
Creative Elements:
- Descriptive Language: The narrative used vivid descriptions like “serene symphony of electric motors” and “smog-filled streets of the past.”
- Comprehensive Vision: The story incorporated all requested elements (urban planning, air quality, energy infrastructure, economic impacts) within a cohesive narrative.
- Balanced Perspective: The story acknowledged both benefits and remaining challenges in this future scenario.
The story was completed in 43 seconds, with 543 words written at a 12th-grade reading level.
What This Tells Us About Gemini 2.0 Flash-Lite
Based on these performance examples, several key characteristics emerge:
- Speed Without Sacrificing Quality: The model completed complex tasks in seconds while maintaining high output quality.
- Multi-Step Reasoning: For calculation and comparison tasks, the model demonstrated the ability to break problems into logical steps.
- Research Integration: The content generation task showed how the model can gather information from multiple sources and synthesize it effectively.
- Format Flexibility: The model adapted its output style from analytical (comparison) to creative (narrative) based on requirements.
For users and developers, this suggests that Gemini 2.0 Flash-Lite represents a solid balance between performance speed and output quality, making it potentially valuable for tasks ranging from content creation to data analysis and creative work.
Looking Forward
As AI systems continue to evolve, the balance between processing speed and output quality becomes increasingly important. Models like Gemini 2.0 Flash-Lite demonstrate how even faster, lightweight AI systems can handle diverse tasks effectively.
For businesses and individuals considering AI assistance for tasks like content creation, data analysis, or creative projects, these performance indicators provide useful benchmarks for what’s possible with current technology.
What tasks would you use a system like this for? The versatility demonstrated across these examples suggests numerous practical applications across professional, educational, and personal contexts.