In today’s rapidly evolving AI landscape, model performance benchmarking provides crucial insights for developers, researchers, and business decision-makers. Google’s Gemini 2.0 Thinking represents an intriguing experimental model that exposes its reasoning process. This analysis examines its capabilities across five fundamental task categories, providing a detailed breakdown of its strengths, limitations, and practical applications.
Methodology
Our evaluation methodology involved testing Gemini 2.0 Thinking on five representative task types:
- Content Generation – Creating structured informational content
- Calculation – Solving multi-step mathematical problems
- Summarization – Condensing complex information efficiently
- Comparison – Analyzing and contrasting complex topics
- Creative/Analytical Writing – Producing detailed scenario analyses
For each task, we measured:
- Processing time
- Output quality
- Reasoning approach
- Tool utilization patterns
- Readability metrics
Task 1: Content Generation Performance
Task Description: Generate a comprehensive article about project management fundamentals, focusing on defining objectives, scope, and delegation.
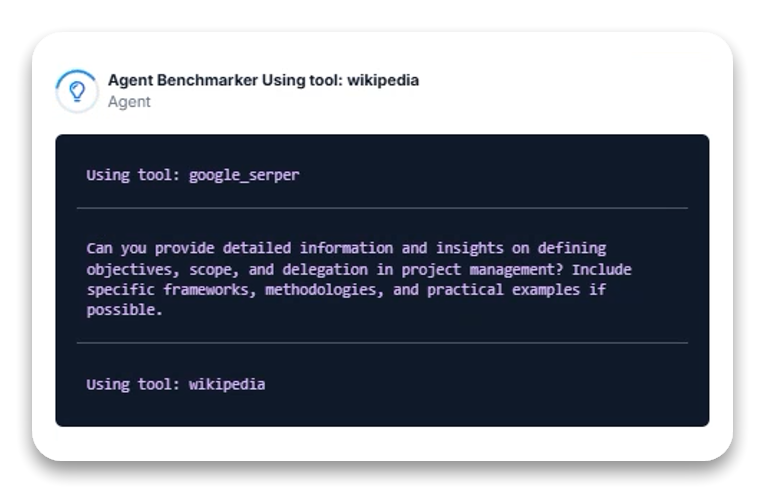
Performance Analysis:
What makes Gemini 2.0 Thinking particularly noteworthy is its visible reasoning process. The model demonstrated a systematic, multi-stage research and synthesis approach across two task variants:
- Starting with Wikipedia for foundational context
- Using Google Search for specific details and best practices
- Further refining searches based on initial findings
- Crawling specific URLs for deeper information
Information Processing Strengths:
- In the second variant, the model demonstrated advanced source identification, crawling multiple URLs to extract detailed information
- Created highly structured outputs with clear hierarchical organization (13 grade reading level)
- Successfully incorporated specific frameworks requested in the prompt (SMART, OKRs, WBS, RACI Matrix)
- Effectively balanced theoretical concepts with practical applications
Efficiency Metrics:
- Processing times: 30 seconds (Task Variant 1) vs. 56 seconds (Task Variant 2)
- The longer processing time in Variant 2 corresponds with more extensive research (additional URL crawling) and a more detailed output (710 words vs. ~500 in Variant 1)
Performance Rating: 9/10
The content generation performance earns a high rating due to the model’s ability to:
- Conduct multi-source research autonomously
- Structure information logically with appropriate headings/subheadings
- Balance theory with practical frameworks
- Adapt research depth based on prompt specificity
- Generate professional-grade content quickly (under 1 minute)
The main strength of the Thinking version is the visibility into its research approach, showing the specific tools used at each stage of the process, though explicit reasoning statements were inconsistently displayed.
Task 2: Calculation Performance
Task Description: Solve a multi-part business calculation problem involving revenue, profit, and optimization.
Performance Analysis:
Across both task variants, the model demonstrated strong mathematical reasoning capabilities:
- Decomposition: Broke complex problems into logical sub-calculations (revenue by product → total revenue → cost by product → total cost → profit by product → total profit)
- Optimization: In the first variant, when asked to determine additional units needed for a 10% revenue increase, the model explicitly stated its optimization approach (prioritizing higher-priced products to minimize total units)
- Verification: In the second variant, the model demonstrated result verification by calculating if the proposed solution (12 units of A, 8 units of B) would achieve the required additional revenue
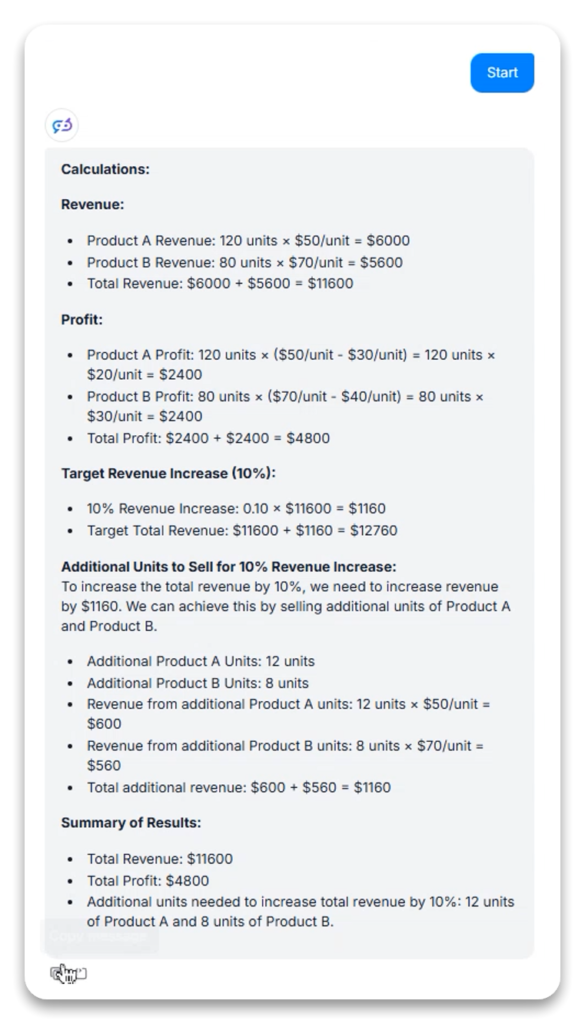
Mathematical Processing Strengths:
- Precision in calculations with no mathematical errors
- Transparent step-by-step breakdown making verification easy
- Effective use of formatting (bullet points, clear section headings) to organize calculation steps
- Different solution approaches between variants showing flexibility in problem-solving
Efficiency Metrics:
- Processing times: 19 seconds (Task Variant 1) vs. 23 seconds (Task Variant 2)
- Consistent performance across both variants despite different solution approaches
Performance Rating: 9.5/10
The calculation performance earns an excellent rating based on:
- Perfect calculation accuracy
- Clear step-by-step process documentation
- Multiple solution approaches demonstrating flexibility
- Efficient processing time
- Effective result presentation and verification
The “Thinking” capability was particularly valuable in the first variant, where the model explicitly outlined its assumptions and optimization strategy, offering transparency into its decision-making process that would be missing in standard models.
Task 3: Summarization Performance
Task Description: Summarize key findings from an article on AI reasoning in 100 words.
Performance Analysis:
The model demonstrated remarkable efficiency in text summarization across both task variants:
- Processing Speed: Completed the summarization in approximately 3 seconds in both variants
- Length Constraint Adherence: Generated summaries well within the 100-word limit (70-71 words)
- Content Selection: Successfully identified and included the most significant aspects of the source text
- Information Density: Maintained high information density while keeping the summary coherent
Summarization Strengths:
- Exceptional processing speed (3 seconds)
- Perfect adherence to length constraints
- Preservation of key technical concepts
- Maintenance of logical flow despite significant compression
- Balanced coverage across source document sections
Efficiency Metrics:
- Processing time: ~3 seconds in both variants
- Summary length: 70-71 words (well within 100-word limit)
- Information compression ratio: Approximately 85-90% reduction from source
Performance Rating: 10/10
The summarization performance earns a perfect rating due to:
- Extraordinarily fast processing time
- Perfect constraint adherence
- Excellent information prioritization
- Strong coherence despite high compression
- Consistent performance across both test variants
Interestingly, for this task, the “Thinking” feature didn’t display explicit reasoning, suggesting the model might engage different cognitive pathways for different tasks, with summarization potentially being more intuitive than stepwise.
Task 4: Comparison Task Performance
Task Description: Compare the environmental impact of electric vehicles with hydrogen-powered cars across multiple factors.
Performance Analysis:
The model demonstrated different approaches across the two variants, with notable differences in processing time and source utilization:
- Variant 1: Relied primarily on Google Search, completed in 20 seconds
- Variant 2: Used Google Search followed by URL crawling for deeper information, completed in 46 seconds
Comparative Analysis Strengths:
- Well-structured comparison frameworks with clear categorical organization
- Balanced perspective on both technologies’ advantages and limitations
- Integration of specific data points (efficiency percentages, refueling times)
- Appropriate technical depth (14-15 grade reading level)
- In Variant 2, proper attribution of information source (Earth.org article)
Information Processing Differences:
- Variant 1 output (461 words) vs. Variant 2 output (362 words)
- Variant 2 showed stronger evidence of specific source utilization
- Both maintained similar readability levels (14-15 grade level)
Performance Rating: 8.5/10
The comparison task performance earns a strong rating due to:
- Well-structured comparative frameworks
- Balanced analysis of advantages/disadvantages
- Technical accuracy and appropriate depth
- Clear organization by relevant factors
- Adaptation of research strategy based on information needs
The “Thinking” capability was evident in the tool usage logs, showing the model’s sequential approach to information gathering: searching broadly first, then specifically targeting URLs for deeper information. This transparency helps users understand the sources informing the comparison.
Task 5: Creative/Analytical Writing Performance
Task Description: Analyze environmental changes and societal impacts in a world where electric vehicles have fully replaced combustion engines.
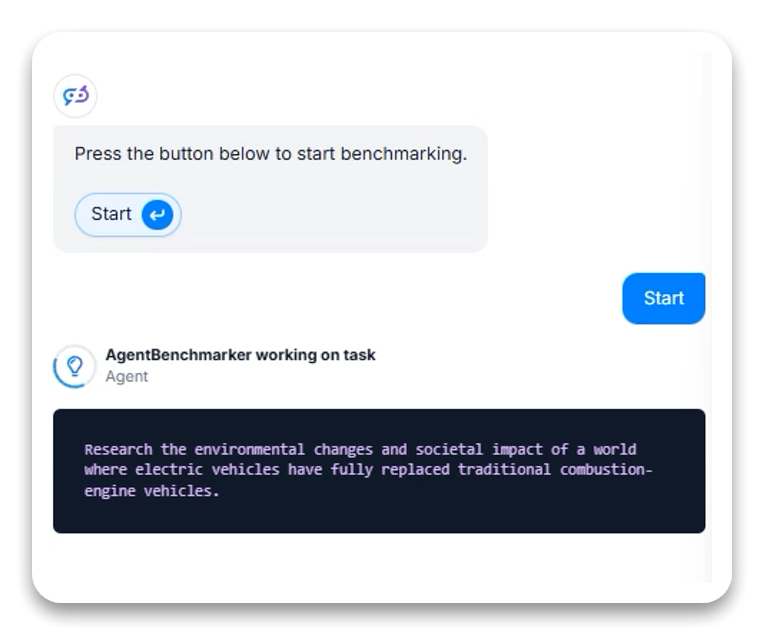
Performance Analysis:
Across both variants, the model demonstrated strong analytical capabilities without visible tool usage:
- Comprehensive Coverage: Addressed all requested aspects (urban planning, air quality, energy infrastructure, economic impact)
- Structural Organization: Created well-organized content with logical flow and clear section headings
- Nuanced Analysis: Considered both benefits and challenges, providing a balanced perspective
- Interdisciplinary Integration: Successfully connected environmental, social, economic, and technological factors
Content Generation Strengths:
- Appropriate tone adaptation (slightly conversational framing in Variant 2)
- Exceptional output length and detail (1829 words in Variant 2)
- Strong readability metrics (12-13 grade reading level)
- Inclusion of nuanced considerations (equity concerns, implementation challenges)
Efficiency Metrics:
- Processing times: 43 seconds (Variant 1) vs. 39 seconds (Variant 2)
- Word counts: ~543 words (Variant 1) vs. 1829 words (Variant 2)
Performance Rating: 9/10
The creative/analytical writing performance earns an excellent rating based on:
- Comprehensive coverage of all requested aspects
- Impressive output length and detail
- Balance between optimistic vision and pragmatic challenges
- Strong interdisciplinary connections
- Fast processing despite complex analysis
For this task, the “Thinking” aspect was less evident in the visible logs, suggesting the model may rely more on internal knowledge synthesis rather than external tool utilization for creative/analytical tasks.
Overall Performance Assessment
Based on our comprehensive evaluation, Gemini 2.0 Thinking demonstrates impressive capabilities across diverse task types, with its distinguishing feature being the visibility into its problem-solving approach:
Task Type | Score | Key Strengths | Areas for Improvement |
---|---|---|---|
Content Generation | 9/10 | Multi-source research, structural organization | Consistency in reasoning display |
Calculation | 9.5/10 | Precision, verification, step clarity | Full reasoning display across all variants |
Summarization | 10/10 | Speed, constraint adherence, information prioritization | Transparency into selection process |
Comparison | 8.5/10 | Structured frameworks, balanced analysis | Consistency in approach, processing time |
Creative/Analytical | 9/10 | Coverage breadth, detail depth, interdisciplinary connections | Tool usage transparency |
Overall | 9.2/10 | Processing efficiency, output quality, process visibility | Reasoning consistency, tool selection clarity |
The “Thinking” Advantage
What distinguishes Gemini 2.0 Thinking from standard AI models is its experimental approach to exposing internal processes. Key advantages include:
- Tool Usage Transparency – Users can see when and why the model employs specific tools like Wikipedia, Google Search, or URL crawling
- Reasoning Glimpses – In some tasks, particularly calculations, the model explicitly shares its reasoning process and assumptions
- Sequential Problem-Solving – The logs reveal the model’s sequential approach to complex tasks, showing how it builds understanding progressively
- Research Strategy Insight – The visible process demonstrates how the model refines searches based on initial findings
This transparency offers several benefits:
- Increased trust through process visibility
- Educational value in observing expert-level problem-solving
- Debugging potential when outputs don’t meet expectations
- Research insights into AI reasoning patterns
Practical Applications
Gemini 2.0 Thinking shows particular promise for applications requiring:
- Research and Synthesis – The model efficiently gathers and organizes information from multiple sources
- Educational Demonstrations – Its visible reasoning process makes it valuable for teaching problem-solving approaches
- Complex Analysis – Strong capability in interdisciplinary reasoning with transparent methodology
- Collaborative Work – The reasoning transparency allows humans to better understand and build upon the model’s work
The model’s combination of speed, quality, and process visibility makes it particularly suitable for professional contexts where understanding the “why” behind AI conclusions is as important as the conclusions themselves.
Conclusion
Gemini 2.0 Thinking represents an interesting experimental direction in AI development, focusing not just on output quality but on process transparency. Its performance across our test suite demonstrates strong capabilities in research, calculation, summarization, comparison, and creative/analytical writing tasks, with particularly exceptional results in summarization (10/10).
The “Thinking” approach provides valuable insights into how the model tackles different problems, though the level of transparency varies significantly between task types. This inconsistency represents the primary area for improvement, as greater uniformity in reasoning display would enhance the model’s educational and collaborative value.
Overall, with a composite score of 9.2/10, Gemini 2.0 Thinking stands as a highly capable AI system with the added benefit of process visibility, making it particularly suitable for applications where understanding the reasoning pathway is as important as the final output.