This first stat may be from last year, but it couldn’t be more relevant today. According to KPMG’s 2024 U.S. CEO Outlook, a striking 68% of CEOs identified AI as a top investment priority. They’re counting on it to boost efficiency, upskill their workforce, and fuel innovation across their organizations.
That’s a huge vote of confidence in AI — but it also raises an important question: with so much at stake, how do organizations ensure they are using AI responsibly and ethically?
This is where the KPMG AI Risk and Controls Guide comes in. It offers a clear, practical framework to help businesses embrace AI’s potential while managing the real risks it brings. In today’s landscape, building trustworthy AI isn’t just good practice — it’s a business imperative.
Artificial Intelligence (AI) is revolutionizing industries, unlocking new levels of efficiency, innovation, and competitiveness. Yet with this transformation comes a distinct set of risks and ethical challenges that organizations must manage carefully to maintain trust and ensure responsible use. The KPMG AI Risk and Controls Guide is designed to support organizations in navigating these complexities, providing a practical, structured, and values-driven approach to AI governance.
Aligned with KPMG’s Trusted AI Framework, this guide helps businesses develop and deploy AI solutions that are ethical, human-centric, and compliant with global regulatory standards. It is organized around 10 foundational pillars, each addressing a critical aspect of AI risk management:
- Accountability: Clear responsibility for AI outcomes.
- Fairness: Reducing bias and promoting equitable outcomes.
- Transparency: Making AI processes understandable and visible.
- Explainability: Providing reasons behind AI decisions.
- Data Integrity: Ensuring high-quality, reliable data.
- Reliability: Delivering consistent and accurate performance.
- Security: Protecting AI systems from threats and vulnerabilities.
- Safety: Designing systems to prevent harm and mitigate risks.
- Privacy: Safeguarding personal and sensitive data.
- Sustainability: Minimizing environmental impacts of AI systems.
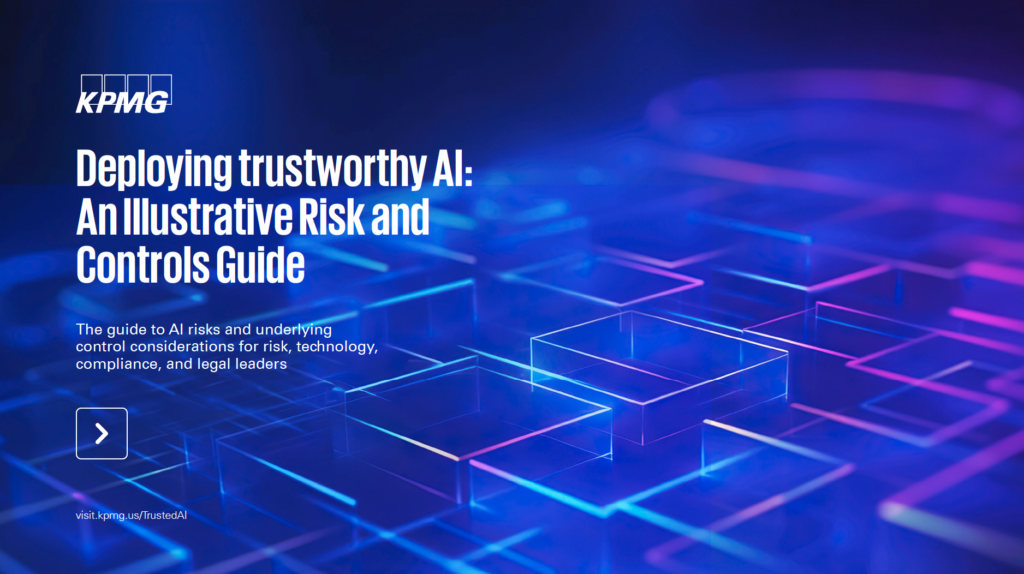
By focusing on these pillars, organizations can embed ethical principles into every phase of the AI lifecycle—from strategy and development to deployment and monitoring. This guide not only enhances risk resilience but also fosters innovation that is sustainable, trustworthy, and aligned with societal expectations.
Whether you are a risk professional, executive leader, data scientist, or legal advisor, this guide provides essential tools and insights to help you responsibly harness the power of AI.
Purpose of the Guide
Addressing the Unique Challenges of AI
The KPMG AI Risk and Controls Guide serves as a specialized resource to help organizations manage the specific risks linked to artificial intelligence (AI). It acknowledges that while AI offers significant potential, its complexities and ethical concerns require a focused approach to risk management. The guide provides a structured framework to tackle these challenges in a responsible and effective manner.
Integration into Existing Frameworks
The guide is not intended to replace current systems but is designed to complement existing risk management processes. Its main goal is to incorporate AI-specific considerations into an organization’s governance structures, ensuring smooth alignment with current operational practices. This approach allows organizations to strengthen their risk management capabilities without needing to completely redesign their frameworks.
Alignment with Trusted Standards
The guide is built on KPMG’s Trusted AI framework, which promotes a values-driven and human-centered approach to AI. It integrates principles from widely respected standards, including ISO 42001, the NIST AI Risk Management Framework, and the EU AI Act. This ensures the guide is both practical and aligned with globally recognized best practices and regulatory requirements for AI governance.
A Toolkit for Actionable Insights
The guide offers actionable insights and practical examples tailored to address AI-related risks. It encourages organizations to adapt these examples to their specific contexts, considering variables like whether the AI systems are developed in-house or by vendors, as well as the types of data and techniques used. This adaptability ensures the guide remains relevant for various industries and AI applications.
Supporting Ethical and Transparent AI Deployment
The guide focuses on enabling organizations to deploy AI technologies in a safe, ethical, and transparent manner. By addressing the technical, operational, and ethical aspects of AI risks, it helps organizations build trust among stakeholders while leveraging AI’s transformative capabilities.
The guide acts as a resource to ensure AI systems align with business objectives while mitigating potential risks. It supports innovation in a way that prioritizes accountability and responsibility.
Who Should Use This Guide?
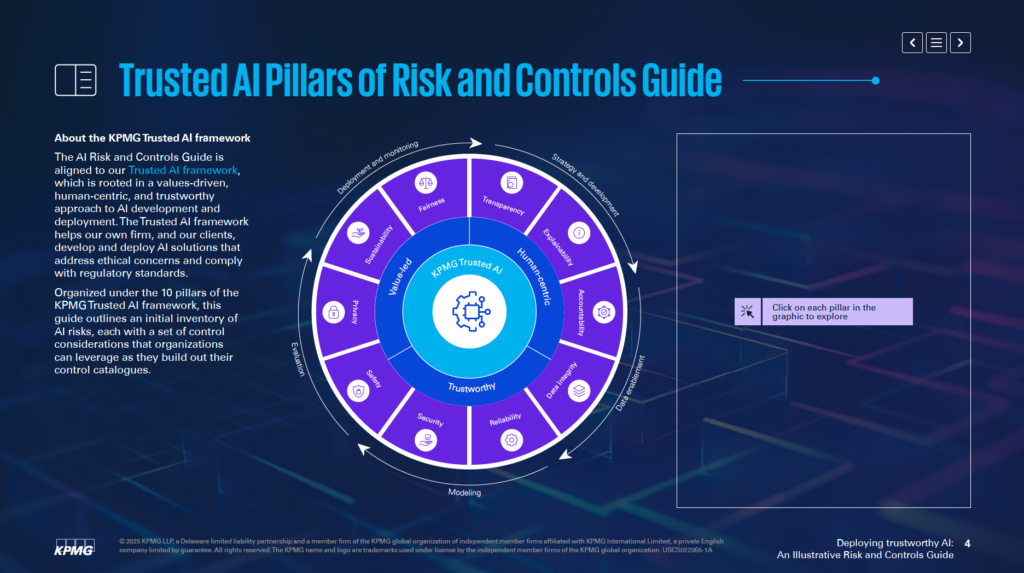
Key Stakeholders in AI Governance
The KPMG AI Governance Guide is designed for professionals managing AI implementation and ensuring it is deployed safely, ethically, and effectively. It applies to teams across various areas within organizations, including:
- Risk and Compliance Departments: Professionals in these teams can align AI governance practices with current risk frameworks and regulatory requirements.
- Cybersecurity Specialists: With the growing risk of adversarial attacks on AI systems, cybersecurity teams can use this guide to establish strong security measures.
- Data Privacy Teams: The guide provides tools for data privacy officers to manage sensitive information responsibly while addressing compliance concerns related to personal data.
- Legal and Regulatory Teams: Legal professionals can rely on the guide’s alignment with global frameworks, such as GDPR, ISO 42001, and the EU AI Act, to ensure AI systems comply with applicable laws.
- Internal Audit Professionals: Auditors can use the guide to assess whether AI systems meet ethical and operational standards effectively.
Leadership and Strategic Decision-Makers
C-suite executives and senior leaders, such as CEOs, CIOs, and CTOs, will find this guide helpful for managing AI as a strategic priority. According to KPMG’s 2024 US CEO Outlook, 68% of CEOs consider AI a key investment area. This guide enables leadership to align AI strategies with organizational objectives while addressing associated risks.
AI Developers and Engineers
Software engineers, data scientists, and others responsible for creating and deploying AI solutions can use the guide to incorporate ethical principles and robust controls directly into their systems. It focuses on adapting risk management practices to the specific architecture and data flows of AI models.
Organizations of All Sizes and Sectors
The guide is adaptable for businesses developing AI systems in-house, sourcing them from vendors, or using proprietary datasets. It is especially relevant for industries such as finance, healthcare, and technology, where advanced AI applications and sensitive data are critical to operations.
Why This Guide Matters
Deploying AI without a clear governance framework can lead to financial, regulatory, and reputational risks. The KPMG guide works with existing processes to provide a structured, ethical approach to managing AI. It promotes accountability, transparency, and ethical practices, helping organizations use AI responsibly while unlocking its potential.
Getting Started with the Guide
Aligning AI Risks with Existing Risk Taxonomy
Organizations should start by linking AI-specific risks to their current risk taxonomy. A risk taxonomy is a structured framework used to identify, organize, and address potential vulnerabilities. Since AI introduces unique challenges, traditional taxonomies need to expand to include AI-specific factors. These factors might involve data flow accuracy, the logic behind algorithms, and the reliability of data sources. By doing this, AI risks become part of the organization’s broader risk management efforts rather than being treated separately.
The guide points out the need to assess the entire lifecycle of AI systems. Important areas to examine include where data originates, how it moves through processes, and the foundational logic of the AI model. Taking this broad view helps you pinpoint where vulnerabilities may occur during the development and use of AI.
Tailoring Controls to Organizational Needs
AI systems differ based on their purpose, development methods, and the type of data they use. Whether a model is created in-house or obtained from an external provider greatly affects the risks involved. Similarly, the kind of data—whether proprietary, public, or sensitive—along with the techniques used to build the AI, requires customized risk management strategies.
The guide suggests adapting control measures to match the specific needs of your AI systems. For example, if you rely on proprietary data, you may need stricter access controls. On the other hand, using an AI system from a vendor might call for in-depth third-party risk assessments. By tailoring these controls, you can address the specific challenges of your AI systems more effectively.
Embedding Risk Considerations Across the AI Lifecycle
The guide recommends incorporating risk management practices throughout every stage of the AI lifecycle. This includes planning for risks during the design phase, setting up strong monitoring systems during deployment, and regularly updating risk evaluations as the AI system evolves. By addressing risks at each step, you can reduce vulnerabilities and ensure that your AI systems are both ethical and reliable.
Taking the initial step of aligning AI risks with your existing risk taxonomy and customizing controls based on your needs helps establish a solid foundation for trustworthy AI. These efforts enable organizations to systematically identify, evaluate, and manage risks, building a strong framework for AI governance.
The 10 Pillars of Trustworthy AI
The KPMG Trusted AI Framework is built on ten key pillars that address the ethical, technical, and operational challenges of artificial intelligence. These pillars guide organizations in designing, developing, and deploying AI systems responsibly, ensuring trust and accountability throughout the AI lifecycle.
Accountability
Human oversight and responsibility should be part of every stage of the AI lifecycle. This means defining who is responsible for managing AI risks, ensuring compliance with laws and regulations, and maintaining the ability to intervene, override, or reverse AI decisions if needed.
Fairness
AI systems should aim to reduce or eliminate bias that could negatively impact individuals, communities, or groups. This involves carefully examining data to ensure it represents diverse populations, applying fairness measures during development, and continuously monitoring outcomes to promote equitable treatment.
Transparency
Transparency requires openly sharing how AI systems work and why they make specific decisions. This includes documenting system limitations, performance results, and testing methods. Users should be notified when their data is being collected, AI-generated content should be clearly labeled, and sensitive applications like biometric categorization must provide clear user notifications.
Explainability
AI systems must provide understandable reasons for their decisions. To achieve this, organizations should document datasets, algorithms, and performance metrics in detail, enabling stakeholders to analyze and reproduce results effectively.
Data Integrity
The quality and reliability of data during its entire lifecycle—collection, labeling, storage, and analysis—are essential. Controls should be in place to address risks like data corruption or bias. Regularly checking data quality and performing regression tests during system updates helps maintain the accuracy and reliability of AI systems.
Privacy
AI solutions must follow privacy and data protection laws. Organizations need to handle data subject requests properly, conduct privacy impact assessments, and use advanced methods like differential privacy to balance data usability with protecting individuals’ privacy.
Reliability
AI systems should perform consistently according to their intended purpose and required accuracy. This requires thorough testing, mechanisms to detect anomalies, and continuous feedback loops to validate system outputs.
Safety
Safety measures protect AI systems from causing harm to individuals, businesses, or property. These measures include designing fail-safes, monitoring for issues like data poisoning or prompt injection attacks, and ensuring systems align with ethical and operational standards.
Security
Strong security practices are necessary to protect AI systems from threats and malicious activities. Organizations should conduct regular audits, perform vulnerability assessments, and use encryption to safeguard sensitive data.
Sustainability
AI systems should be designed to minimize energy use and support environmental goals. Sustainability considerations should be included from the beginning of the design process, with ongoing monitoring of energy consumption, efficiency, and emissions throughout the AI lifecycle.
By following these ten pillars, organizations can create AI systems that are ethical, trustworthy, and aligned with societal expectations. This framework provides a clear structure for managing AI challenges while promoting responsible innovation.

Key Risks and Controls – Data Integrity
Data Integrity in AI Systems
Data integrity is critical for ensuring AI systems remain accurate, fair, and reliable. Poor data management can lead to risks like bias, inaccuracy, and unreliable results. These issues can weaken trust in AI outputs and cause major operational and reputational problems. The KPMG Trusted AI framework highlights the need to maintain high-quality data throughout its lifecycle to ensure AI systems function effectively and meet ethical standards.
Key Risks in Data Integrity
Lack of Data Governance
Without strong data governance, AI systems may produce flawed results. Issues such as incomplete, inaccurate, or irrelevant data can lead to biased or unreliable outputs, increasing risks across different AI applications.
Data Corruption During Transfers
Data often moves between systems for activities like training, testing, or operations. If these transfers are not handled properly, data may become corrupted, lost, or degraded. This can impact how AI systems perform.
Control Measures to Reduce Risks
Developing Comprehensive Data Governance Policies
To improve data governance, organizations can:
- Create and enforce policies for data collection, storage, labeling, and analysis.
- Implement lifecycle management processes to keep data accurate, complete, and relevant.
- Perform regular quality checks to quickly identify and fix any issues.
Protecting Data Transfers
To minimize risks during data transfers, organizations should:
- Use secure protocols to prevent corruption or loss of data.
- Regularly review training and testing datasets, especially during system updates, to ensure they remain adequate and relevant. This includes adding new data when needed to maintain system performance.
Ongoing Monitoring and Validation
Using continuous monitoring systems helps maintain data integrity throughout the AI lifecycle. These systems can detect problems such as unexpected changes in dataset quality or inconsistencies in data handling. This allows for quick corrective actions when issues arise.
Conclusion
Maintaining data integrity is essential for deploying trustworthy AI systems. Organizations can reduce risks by establishing strong governance frameworks, protecting data interactions, and maintaining continuous validation processes. These actions improve the reliability of AI outputs while ensuring ethical and operational standards are met, helping build trust in AI technologies.
Key Risks and Controls – Privacy
Data Subject Access Privacy
Managing requests related to data subject access is a major privacy challenge in AI. Organizations must make sure individuals can exercise their rights to access, correct, or delete personal information under laws like GDPR and CCPA. If these requests are not handled properly, it may lead to violations of regulations, a loss of consumer trust, and harm to the organization’s reputation.
To reduce this risk, companies should create programs to educate individuals about their data rights when interacting with AI. Systems must be set up to process these requests quickly and transparently. Organizations should also keep detailed records of how they handle these requests to prove compliance during audits.
Privacy Violations from Data Breaches
AI systems often handle sensitive personal information, which makes them attractive targets for cyberattacks. If a breach occurs, it can cause significant regulatory fines, damage to a company’s reputation, and a loss of customer trust.
To combat this, the KPMG Trusted AI framework suggests conducting ethical reviews for AI systems that use personal data to ensure they meet privacy regulations. Regular data protection audits and privacy impact assessments (PIAs) are also necessary, especially when sensitive data is used for tasks like training AI models. Additionally, methods such as differential privacy, which adds statistical noise to data, can help anonymize information while still allowing for analysis.
Lack of Privacy by Design
AI systems that do not include privacy safeguards from the start can create serious issues. Without applying privacy-by-design principles, organizations risk exposing sensitive data or failing to comply with legal requirements.
Companies should include privacy measures during the development stages of AI systems. This involves following privacy laws and data protection regulations through strong data management practices. Clear documentation of how data is collected, used, and stored is crucial. Organizations must also get explicit user consent for data collection and processing, especially in sensitive areas like biometric data.
Transparency in User Interactions
When AI systems do not clearly explain how user data is handled, it can result in mistrust and legal scrutiny. Users should know when their data is collected and how it is being used.
To address this, companies should label AI-generated content clearly and inform users about data collection practices. Giving users the option to opt out of data collection allows them to maintain control over their information. Organizations should also explain the limitations and possible inaccuracies of AI outputs to build trust and encourage users to critically assess the system’s results.
Building a Culture of Privacy
Creating a culture that prioritizes privacy helps organizations manage AI-related risks effectively. Companies should take a values-based approach, incorporating privacy considerations into every phase of the AI lifecycle. This includes training employees on privacy practices, regularly updating privacy policies to meet changing regulations, and aligning with frameworks like GDPR, CCPA, and the KPMG Trusted AI framework.
By addressing privacy risks proactively, organizations can build trust in their AI systems, reduce regulatory risks, and protect customer relationships.
Key Risks and Controls – Safety
The Importance of Addressing AI Safety Risks
AI systems bring significant advancements but also carry various safety risks. These can arise from unexpected behaviors, uncorrected errors, or external threats. Such risks are especially serious in areas like healthcare, autonomous vehicles, and financial systems. If not managed, they can cause system failures, security breaches, or harm to individuals and organizations.
AI System Errors and Their Impact
Errors in AI systems, if left unaddressed, can disrupt operations, resulting in unauthorized changes, system failures, or data loss. Many of these issues happen because there is no strong framework to monitor and resolve errors. For instance, if an AI system in a financial institution misinterprets data, it might carry out incorrect transactions or enable fraudulent activities.
Controls for Mitigating Errors
- Periodic Decision Reviews: Regularly examine a sample of decisions made by AI to confirm they meet business goals and follow ethical guidelines.
- Anomaly Detection Systems: Use systems to spot unusual activity, such as prompt injection attacks or data poisoning, that could undermine the AI’s reliability.
- Feedback Loops: Create processes to continuously check AI outputs and minimize the risk of harmful or incorrect results.
Preventing Harmful AI Outputs
Generative AI models sometimes produce unreliable or harmful content, such as biased or factually incorrect information. This issue, often referred to as “AI hallucinations,” can damage trust and harm reputations.
Controls for Content Management
- Validation Mechanisms: Set up systems to regularly monitor and verify AI outputs.
- Human Moderation: Assign people to review and address reports of misuse or inaccuracies in the AI-generated content.
The Role of Human Oversight in AI Safety
Without human involvement, AI systems can become more prone to risks, especially in critical situations. Systems lacking oversight may not respond effectively to unexpected events.
Controls to Ensure Oversight
- Intervention Policies: Develop clear guidelines for human intervention, so staff can override or reverse AI decisions when necessary.
- Transparency in AI Usage: Clearly communicate with operators and users about the limitations and potential errors in AI decisions to maintain accountability.
Enhancing AI Safety with Proactive Monitoring
Proactively monitoring AI systems helps detect and address problems early. Real-time tracking can reveal performance issues and allow for timely corrections.
Key Monitoring Practices
- Real-Time AI Audits: Regularly inspect AI systems to find and fix vulnerabilities.
- Environmental Impact Tracking: Keep track of the energy usage and efficiency of AI systems to meet sustainability goals while maintaining safety.
By applying these controls to their AI systems, organizations can lower safety risks and improve the dependability of AI technologies. Strong safety measures not only comply with regulations but also build trust in AI-driven solutions.
Key Risks and Controls – Sustainability
Environmental Impact of AI Systems
AI systems, especially large-scale ones, use a lot of energy throughout their lifecycle. Training advanced models like GPT-4 requires extensive computing power, which increases electricity usage, carbon dioxide emissions, and stresses energy grids. Additionally, cooling AI servers consumes significant amounts of water, which can deplete local water supplies and harm ecosystems.
Resource Extraction and Waste Generation
AI depends on high-performance hardware, which requires rare earth elements and minerals. These materials are often mined using unsustainable methods, leading to environmental damage. The process also generates electronic waste containing harmful substances, which can pose serious risks to both human health and the environment.
Controls to Mitigate Sustainability Risks
1. Set Sustainability Goals Early
Organizations should establish clear sustainability goals during the development of AI systems. These goals should align with broader environmental commitments to ensure the systems are designed to operate efficiently with minimal environmental harm.
2. Continuous Monitoring of Energy and Resource Use
Using real-time tracking tools can help monitor energy consumption, efficiency, and emissions from AI systems. These tools allow organizations to evaluate whether they are meeting sustainability targets and make adjustments if they are not.
3. Adopt Green AI Practices
Organizations can use “Green AI” methods to reduce environmental impacts. These methods include optimizing algorithms to use fewer computational resources and powering data centers with renewable energy.
4. Promote Circular Economy in AI Hardware
To reduce electronic waste, organizations should focus on recycling and reusing AI hardware. Building systems with modular components can make it easier to upgrade hardware sustainably and minimize waste.
Balancing AI Innovation and Sustainability
AI has the potential to address global environmental issues, but its development must avoid harming the planet. By including sustainability principles in AI governance, organizations can create systems that are both innovative and environmentally sustainable.
Key Risks and Controls – Transparency
Addressing AI Transparency Challenges
Transparency is essential for building trust in AI systems. It deals with the “black box” issue, where AI systems function in ways that are not easily understood. This lack of clarity can lead to trust issues, ethical concerns, and compliance risks. To address these problems, organizations need to implement clear strategies to enhance transparency throughout the AI lifecycle.
Responsible Disclosure and Documentation
AI systems should include mechanisms for responsible disclosure to provide stakeholders with clear insights into how the system works. Key strategies include:
- Detailed Documentation of Limitations and Metrics: Developers should record the system’s limitations, performance metrics, and testing methods. This helps internal teams understand the system’s capabilities and risks, enabling informed decisions during deployment.
- Explainable AI (XAI) Techniques: These methods make AI decision-making more understandable. When IT teams and other relevant personnel can interpret the system’s processes, they can manage it with greater confidence.
- Transparency in Testing and Validation: Organizations should keep detailed records of test datasets, performance results, and validation tools. This ensures that testing processes are consistent and that results can be reproduced.
User Transparency and Awareness
Transparency also needs to extend to users who interact with AI systems. Suggested measures include:
- Clear Identification of AI Content: AI-generated outputs should be clearly labeled or watermarked to distinguish them from human-created content, reducing any risk of confusion or deception.
- Notification of Potential Inaccuracies: Users should be informed that AI outputs may contain inaccuracies and should approach the information critically.
- Data Collection Notifications: Organizations must notify users when collecting their data and provide simple options to opt out. For sensitive applications, like biometric data collection, organizations must ensure explicit user consent and pre-deployment notifications.
Helping Stakeholders Understand
Transparency is incomplete if stakeholders, including IT teams and end users, do not fully understand the AI system. Organizations should:
- Educate Stakeholders: Provide training and awareness programs to help stakeholders understand the system’s functions, risks, and ethical considerations.
- Encourage Open Communication: Establish clear reporting and communication channels within the organization to address any uncertainties or concerns related to the AI system.
Building Trust Through Transparency
By embedding transparency-focused measures into AI governance frameworks, organizations can align their systems with ethical guidelines and regulatory requirements. Ensuring that stakeholders have a clear understanding of the system’s purpose, functionality, and limitations helps address risks and supports accountability. Proactively resolving transparency challenges can boost trust and maintain consumer confidence in the organization’s AI solutions.
Practical Examples of Controls
Accountability: Ensuring Ethical AI Alignment
Accountability ensures that AI systems follow organizational ethics and business goals. To put this into practice, organizations can conduct regular assessments of their AI systems. These assessments verify if AI outputs comply with ethical standards, regulatory requirements, and intended objectives. Assigning specific roles, like a Chief AI Officer, can also help maintain oversight of AI systems and ensure accountability.
Fairness: Addressing Bias in AI Systems
Fairness aims to reduce biases during the creation and use of AI systems. For example, organizations can train development teams on bias awareness. This helps teams recognize and minimize biases that could harm various user groups. Another practical step is conducting fairness audits during the design and testing phases of AI models to ensure they are fair and inclusive.
Data Integrity: Maintaining High-Quality AI Inputs
Data integrity ensures reliable AI outputs by keeping input data accurate and relevant. One way to maintain integrity is by performing regression testing during system updates. This testing checks whether updates affect the quality or accuracy of AI outputs. Additionally, organizations can implement data governance frameworks to oversee processes like data collection, labeling, and storage, ensuring high-quality inputs throughout the data lifecycle.
Transparency: Enhancing AI Trustworthiness
Transparency helps address the lack of clarity in how AI systems work. Organizations can make AI-generated content identifiable by adding labels, watermarks, or disclaimers to inform users about its source. Internally, documenting limitations, performance metrics, and testing processes can help educate teams and stakeholders. Another step is allowing users to opt out of data collection and providing clear notifications when sensitive applications, such as biometric categorization, are used.
Privacy: Safeguarding Personal Data
Privacy controls protect user data and ensure compliance with regulations like GDPR. Organizations can apply privacy controls by conducting regular data protection audits and privacy impact assessments (PIAs). Using techniques like differential privacy, which adds statistical noise to datasets, can help anonymize sensitive information while maintaining its usefulness for analysis.
Safety: Preventing AI System Failures
Safety measures reduce risks like system errors or malicious attacks. A practical example is using anomaly detection systems to identify unusual activities, such as data poisoning or prompt injection attacks. Establishing feedback loops to validate AI outputs continuously is another way to ensure they meet predefined safety and ethical standards.
Sustainability: Reducing AI’s Environmental Footprint
Sustainability focuses on minimizing the energy consumption of AI systems. Organizations can set energy efficiency goals during development and use real-time monitoring tools to track energy usage, efficiency, and emissions throughout the AI system’s lifecycle. This helps identify opportunities to improve energy usage and meet environmental commitments.
Operationalizing AI Controls Through Tailored Approaches
These examples show how organizations can apply AI controls effectively by adapting them to fit their specific needs and risks. By integrating these controls into existing governance frameworks, businesses can ensure their AI systems remain ethical, reliable, and aligned with their overall objectives.
KPMG’s Additional Support
KPMG offers a wide range of services to help organizations manage AI risks effectively. These services are designed to address the specific challenges of using AI while ensuring adherence to ethical standards and regulatory requirements.
Trusted AI Strategy
KPMG works with organizations to create strategic plans for using AI responsibly. This involves aligning AI projects with business objectives, ethical guidelines, and regulatory rules to build a sustainable and reliable AI system.
AI Ethics and Governance
To promote ethical AI usage, KPMG develops governance frameworks based on principles like transparency, accountability, and fairness. These frameworks help organizations handle complex ethical issues and set up strong oversight systems.
AI Risk Assessment and Regulatory Compliance
KPMG performs detailed risk assessments to find potential weaknesses in AI systems. These assessments follow global standards such as the NIST AI Risk Management Framework and the EU AI Act, ensuring that systems comply with current regulations.
Machine Learning Operations (MLOps)
Maintaining the performance of AI systems is essential. KPMG provides MLOps solutions to ensure AI models remain efficient throughout their lifecycle, including processes like updates and retraining.
AI Security
Security is a key part of KPMG’s services. They implement methods to protect AI systems from risks such as data breaches, adversarial attacks, and vulnerabilities, ensuring the systems produce reliable and secure outputs.
AI Assurance
KPMG performs independent reviews to verify the performance and reliability of AI systems. This includes auditing models to check for fairness, bias, and regulatory compliance, which helps build trust among stakeholders.
With these services, KPMG supports organizations in implementing the Trusted AI Framework. They make sure that AI systems are ethical, secure, and aligned with business goals. By using KPMG’s expertise, companies can confidently manage the challenges of AI risk management.
Final Thoughts
The Importance of Trust in AI Deployment
Using AI is an essential step for organizations that want to stay competitive. However, while AI offers transformative opportunities, it also comes with significant risks. KPMG’s Trusted AI Framework highlights the need to prioritize trust in every stage of AI development and deployment. By following its ten core principles—accountability, data integrity, explainability, fairness, human oversight, privacy, reliability, safety, security, and transparency—organizations can create AI systems that are both effective and ethically responsible.
Keeping Up with a Rapidly Changing Landscape
AI technology evolves quickly, and so do the risks and challenges it brings. Having a fixed risk management approach is not enough to address these changes. Organizations need to adopt flexible strategies that can adjust as technology advances and new regulations emerge. This ongoing process reduces risks while encouraging innovation by fostering confidence and trust in AI systems.
Making Trust a Collective Effort
Establishing and maintaining trust in AI requires teamwork. This responsibility extends beyond AI developers and governance teams to include data scientists, risk managers, legal experts, and end-users. To operationalize trust, organizations need clear documentation, transparent decision-making, and open communication with users throughout the AI lifecycle.
Deploying AI Responsibly for Sustainable Success
For AI to succeed, its capabilities must align with both organizational goals and societal expectations. Responsible AI deployment goes beyond meeting compliance standards. It involves creating systems that respect human values, protect individual privacy, and support sustainability. This approach not only reduces potential risks but also amplifies the long-term advantages of AI, ensuring it drives meaningful and positive change.
Taking Action
As organizations move forward in integrating AI, the KPMG Trusted AI Framework offers a detailed guide to help manage the complexities of AI-related risks. By embedding these principles into their workflows and adapting them to suit specific needs, businesses can confidently explore AI’s possibilities while maintaining high standards of trust and accountability. The message is clear: adopt ethical, transparent, and adaptable AI practices to fully harness its transformative potential in a responsible and sustainable way.
Title: KPMG AI Risk and Controls Guide
Discover KPMG’s first-of-its-kind AI Risk and Controls Guide, a resource designed to help organizations manage AI risks responsibly and ethically. It provides actionable insights tailored to various industries.
Source URL: https://kpmg.com/us/en/articles/ai-risk-and-control-guide-gated.html
