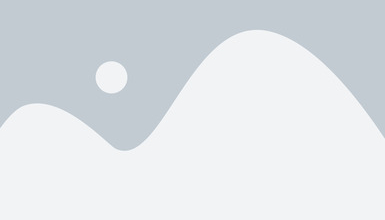
AI Content Creation
AI Content Creation leverages artificial intelligence to automate and enhance digital content generation, curation, and personalization across text, visuals, an...
Content enrichment uses AI to transform unstructured content into structured, insightful data, improving accessibility, search, and business decision-making.
Content Enrichment with AI refers to the process of enhancing raw, unstructured content by applying artificial intelligence techniques to extract meaningful information, structure, and insights. This transformation makes content more accessible, searchable, and useful for various applications such as data analysis, information retrieval, and decision-making.
At its core, content enrichment involves augmenting existing data with additional metadata or context. When combined with AI, this process becomes significantly more powerful. AI algorithms can automatically analyze large volumes of content—text, images, or other data formats—and extract entities, sentiments, topics, and other valuable information without manual intervention.
For example, consider a repository of customer reviews. In their raw form, these reviews are unstructured text that may be challenging to analyze collectively. By applying AI-driven content enrichment, businesses can automatically extract key sentiments, identify trending topics, and categorize feedback based on themes. This enriched data becomes a valuable asset for improving products, services, and customer experiences.
Content Enrichment with AI is utilized across various industries and applications to enhance the value of data. Here are some key ways in which it is used:
AI-powered NLP techniques enable computers to understand and interpret human language. By applying NLP to content enrichment, organizations can extract meaningful information from unstructured text data. This includes:
Use Case Example:
A global company wants to analyze customer feedback from various regions. By using AI for content enrichment, they can automatically detect the language of each feedback entry, translate it into a common language, extract key sentiments, and identify prevalent issues or praises specific to each region.
AI algorithms can process visual content to extract meaningful data from images and videos. This includes:
Use Case Example:
An e-commerce platform wants to improve its product catalog by enriching product images. By applying object detection and OCR, they can automatically identify products, extract text from labels, and categorize items more accurately, enhancing the shopping experience through better search and recommendations.
Businesses often have large datasets that lack context or are incomplete. AI-driven data enrichment provides additional layers of information, such as:
Use Case Example:
A marketing team is planning a targeted campaign. By enriching their customer data with demographic and behavioral information using AI, they can segment the audience effectively, personalize messaging, and increase the campaign’s effectiveness.
Content enrichment improves the quality and relevance of search results by adding structured metadata to unstructured content. This makes information retrieval more efficient and accurate.
Use Case Example:
An enterprise search system within a large organization struggles to deliver relevant documents when employees search for information. By enriching documents with AI-extracted metadata such as topics, author names, dates, and key phrases, the search engine can provide more precise results, improving productivity.
Automated content enrichment assists in identifying sensitive information, ensuring compliance with regulations, and supporting legal discovery processes.
Use Case Example:
A legal team needs to review thousands of documents for a case. AI-powered content enrichment can automatically tag and categorize documents based on relevance, extract key entities, and identify privileged information, significantly reducing the manual workload.
Content enrichment enables chatbots and AI assistants to access enriched data, providing more accurate and contextually relevant responses to user queries.
Use Case Example:
A customer support chatbot uses enriched knowledge bases to answer customer questions more effectively. By accessing content that has been enriched with AI (e.g., FAQs classified by topic, products tagged with detailed attributes), the chatbot can deliver precise answers, improving customer satisfaction.
Scenario:
An organization has a vast collection of unstructured documents, including reports, emails, and memos. They need to extract information about specific entities such as person names, organizations, and locations.
Application:
Using AI-driven named entity recognition, the organization can automatically scan all documents to identify and extract mentions of key entities. This enriched data allows them to:
Scenario:
A media company manages an extensive library of images and videos but lacks detailed metadata, making it difficult to find specific assets.
Application:
By applying AI-powered object detection, they can automatically identify and tag objects within their visual content. For example, tagging images that contain “mountains,” “beach,” or “city skyline.” This enrichment enables:
Scenario:
A retail company collects customer reviews and feedback from multiple channels, including social media, surveys, and support tickets.
Application:
AI-driven sentiment analysis processes the textual feedback to determine the emotional tone—positive, negative, or neutral—of each entry. This enriched data helps the company to:
Scenario:
An online retailer wants to improve the searchability and discoverability of products on their website. The existing product descriptions are incomplete and lack consistency.
Application:
By using AI for product content enrichment, the retailer can:
Benefits:
Scenario:
A finance company needs to enrich its customer data to improve risk assessment models.
Application:
By applying AI to enrich data, the company can:
Outcome:
Scenario:
A knowledge-based organization relies on a content management system (CMS) to store and share documents but faces challenges in content retrieval and classification.
Application:
AI-driven content enrichment processes documents within the CMS to:
Result:
Scenario:
A tech support company uses a chatbot to handle basic customer inquiries but finds that the bot often provides incomplete or irrelevant answers.
Application:
By enriching the underlying knowledge base with AI, the company can:
Impact:
Machine Learning (ML) models learn from data to make predictions or decisions without being explicitly programmed. In content enrichment, ML algorithms can classify content, detect patterns, and make sense of complex data.
Examples:
NLP enables computers to understand, interpret, and generate human language. It is crucial for analyzing unstructured text data.
Components:
Computer Vision allows AI to interpret and understand visual information from the world, such as images or videos.
Applications:
A knowledge graph is a representation of entities and the relationships between them. It provides context and connections between pieces of information.
Use in Content Enrichment:
OCR technology converts different types of documents, such as scanned paper documents or images captured by a digital camera, into editable and searchable data.
Role in Content Enrichment:
Several AI platforms and tools facilitate content enrichment:
Content Enrichment with AI is closely intertwined with AI automation and chatbots:
Content Enrichment with AI is the process of enhancing raw, unstructured content using artificial intelligence to extract meaningful information, add structure, and provide insights, making the content more accessible and useful for various applications.
Businesses use content enrichment to improve data quality, enable advanced analytics, automate document processing, and enhance customer experience through better search, recommendations, and chatbots.
Common techniques include Natural Language Processing (NLP) for text analysis, computer vision for images and videos, entity recognition, sentiment analysis, metadata tagging, and Optical Character Recognition (OCR).
Industries such as healthcare, finance, legal, manufacturing, marketing, and retail benefit from content enrichment by improving search, compliance, decision-making, and customer engagement.
Yes, enriched content improves chatbot performance by providing structured, contextually relevant information, enabling more accurate and helpful responses to user queries.
Discover how Content Enrichment with AI can make your data more valuable, searchable, and actionable for your business.
AI Content Creation leverages artificial intelligence to automate and enhance digital content generation, curation, and personalization across text, visuals, an...
Extractive AI is a specialized branch of artificial intelligence focused on identifying and retrieving specific information from existing data sources. Unlike g...
Research and study better by summarizing the content of URLs with AI. Simply input the URL and get key insights immediately.